A Consideration on Stories of Recent Histories
This story is not a fixed point. This one story here below is neither one that controls all possible AI stories. We are able to note that a history, such as this one, is a handpicking from a source that is richer than the story that is consequentially put in front of you, here, on a linear and engineered chronology. The entirety of the field of AI is more contextualized with parallel storylines, faded-in trials, and faded-out errors, with many small compounded successes and numerous complexities. Histories tend to be messy. This story does not pretend to do justice to that richness.
Just like a numerical dataset, history is a (swirling) pool of data. Just as an information processing unit, hopefully enabled to identify a relevant pattern that still could be prone to an unwanted bias, ambiguities, and probabilities with given uncertainties, so too is this narrative of a history on the dynamic field of AI studies, its researches and its developments. In realizing this, one can only wish that the reader of this story shares the wish to grow towards an increased self-reliant literacy, nurtured with “data” (read the word “data” here as “historical resources” among more numerical sources) and analytical ability.
A Suggested Mini Project Concept for Students:
Mini Project #___ : Datasets & Pattern Recognition Opportunities are Everywhere
The above consideration could be part of any storytelling, and its implication is not an insurmountable problem. It’s an opportunity, especially in the area of data sciences associated with AI research and development. See, this story here as an invitation to go out into the field and study more, to get a more nuanced sense of this history’s depths and its adventures within it. Try to see its datasets and their correlations, fluidities, contradictions and complexities. The ability to do so are essential skills in the field of AI as well as important skills as a human in a complex and changing world.
What “algorithm” might the author of this story here have used when recognizing a pattern from a given dataset in creating this story? (there is no right or wrong answer)
It’s almost obvious that a learner can only aspire toward something they know as something that existed, exists or could be imagined to exist. That is simultaneously through for learning from the data selection processes from another, for instance, the authoring of and the applying of the following history of the field of AI.
Can you create your own version of an AI history? What kind of filter, weight, bias or algorithm have you decided to use in creating your version of an AI history?
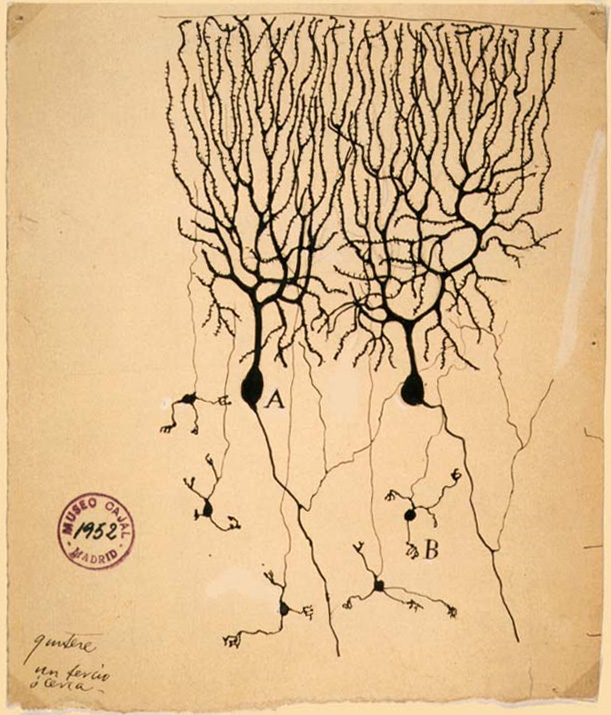
A Recent History of the Field of AI
Just like a present-day AI solution does, a human learner too needs datasets to see their own pattern of their path within the larger field. Who knows, digging into the layers of AI history might spark a drive to innovate on an idea some researchers had touched on in the past yet, have not further developed. This has been known to happen in a number of academic fields of which the field of AI is no exception.[1] Here it is opted to present a recent history of the field of AI[2] with a few milestones from the 20th century and the 21st century:
By the end of the 1930s and during the decade of 1940-1950, scientists and engineers joined with mathematicians, neurologists, psychologists, economists and political scientists to theoretically discuss the development of an artificial brain or of the comparison between the brain, intelligence and what computers could be (note, these did not yet exist in these earliest years of the 1940s).
In 1943, McCulloch & Pitts offered a theoretical proposal for a Boolean logic[3] circuit model of the brain.[4] These could be seen as the theoretical beginnings of what we know today as the Artificial Neural Networks.
In 1950 Turing wrote his seminal paper entitled “Computing Machinery and Intelligence.”[5] Slightly later, in the 1950s, early AI programs included Samuel’s checkers game program, Newell & Simon’s Logic Theorist, or Gelernter’s Geometry Engine. It has been suggested that perhaps the first AI program was the Checkers game software. Games, such as Chess, Wéiqí (aka GO) and others (e.g. LěngPūDàshī, the poker-playing AI[6]) have been, and continue to be, important in the field of AI research and development.
While relatively little about it is said to have sustained the test of time, in 1951, a predecessor of the first Artificial Neural Network was created by Marvin Minsky and Dean Edmonds.[7] It was named “SNARC” which is short for “Stochastic Neural Analog Reinforcement Computer”.[8] The hardware system solved mazes. It simulated a rat finding its way through a maze. This machine was not yet a programmable computer as we know it today.
The academic field of “Artificial Intelligence Research” was created between 1955 and 1956.[9] That year, in 1956, the term “AI” was suggested by John McCarthy. He is claimed to have done so during a Dartmouth College conference that same year in Hanover, New Hampshire, the USA. McCarthy defined AI as “… the science and engineering of making intelligent machines, especially intelligent computer programs. It is related to the similar task of using computers to understand human intelligence, but AI does not have to confine itself to methods that are biologically observable…”[10]
At that same time, the “Logic Theorist” was introduced by other scientists as the first Artificial Intelligence application.[11] It was able to proof a number of mathematical theorems.
In January 1957 Frank Rosenblatt proposed the concept of a single-layered neural network. He invented the photoperceptron (“perceptron” for short), an electronic automaton and model analogous to the brain, in a simplest sense thinkable, that would have the ability to “learn” visual patterns and to process such “…human-like functions as perception, recognition, concept formation, and the ability to generalize from experience… [This system would get its inputs] directly from the physical world rather than requiring the intervention of a human agent to digest and code the necessary information.” [12] In short, the machine was aimed to recognize and classify a given geometric shape, following the input from a camera.
It is natural and healthy in the sciences to inquire with intellectual integrity and wonder, to insist for verification, corroboration and falsifiability[13] of theories and designs. So too did the photoperceptron design not escape scrutiny and the common peer review.[14] At one point, the perceptron was perceived to be of debated applicability and of contested interest as a basis for further research and developments within AI.[15] Several decades later, following a couple of “AI Winters” and academic meanderings, substantial increase in computational power and processing techniques, this will turn out to be a fruitful basis for a specific area of AI research and development: Machine Learning, its area of Deep Learning and its multilayered Artificial Neural Networks.[16]
1959: The term “Machine Learning” was invented by the IBM electrical engineer and Stanford Professor, Arthur Lee Samuel. He wrote the first successful self-learning program. It played a Checkers game.[17] This was an early demonstration of an AI-type application which will become a hot topic in the 21st century and into present-day academic work.
The period from the nineteen fifties (1950s) into the earliest years of the nineteen seventies (early 1970s): during this two-decades-long period there was a lot of excitement around the promises suggested within the research and developments in the Artificial Intelligence field.
In 1965 Robinson invented an algorithm[18] for logical reasoning that laid the groundwork for a form of computer programming,[19] allowing for the automated proving of mathematical theorems.
Around 1970 Minsky and Papert considered the multilayered perceptron (MLP)[20] which could be seen as a theoretical predecessor to the multilayered neural networks as they are researched and developed today, in the AI sub-field of Machine Learning and its Deep Learning techniques.
Reflecting back onto the years around 1973,[21] voices tend to speak of the first “AI Winter”[22] while others don’t seem to mention this period at all.[23] Either way, it means that during this time, it is perceived that two forces supposedly collided: one was that of some academics and other people with a wish to do research in specific directions in the field of AI. They continued needing money. However, other academics with some understandable doubts[24] and those controlling the funds,[25] no longer believed much in the (inflated) promises made within the given AI research of that period in history. Since money as a resource became limited, so too did research and development slow down. More focus and result-oriented work was required to obtain funds. At least, so it seemed for a period of time, until after the mid-seventies or until the early Eighties (1980s).[26] Depending on the historical source this time period has been demarcated rather differently (and perhaps views on what counts as significant might differ).[27]
Fading in from the early 1970s and lasting until the early 1990s, the AI research and developmental focus was on what is referred to as Knowledge-based approaches. Those designing these type of solutions sought to “hard-code knowledge about the world in formal languages…” However, “…none of these projects has led to a major success. One of the most famous such projects is Cyc…”[28] Humans had to physically code the solutions which created a number of concerns and problems. The experts could not sufficiently and accurately code all the nuances of the reality of the world around the topic which the application was supposed to “intelligently” manage.
With the earliest introductions in 1965 by Edward Feigenbaum, one could continue finding further, yet still early, developments of these “Knowledge-based Systems”[29] (KBS). The development of these systems continued into the 1970s, some of which then came to further (commercial) fruition during the 1980s in the form of what was by then called “Expert Systems”(ES). The two systems, KBS and ES, are not exactly the same but they are historically connected. These later systems were claimed to represent how a human expert would think through a highly specific problem. In this case the processing method was conducted by means of IF-THEN rules. During the 1980s the mainstream AI research and development focused on these “Logic-based, Programmed Expert Systems”. Prolog, a programming language, initially aimed at Natural Language Processing,[30] has been one of the favorites in designing Expert Systems.[31] All expert systems are knowledge-based systems, the reverse is not true. By the mid-1980s Professor John McCarthy would criticize these systems as not living up to their promises.[32]
In the late Eighties (late 1980s), Carver Mead[33] introduced the idea to mimic the structure and functions of neuro-biological architecture (e.g. of brains or of the eye’s retina and visual perception) in the research and development of AI solutions (both in hardware and software). This approach (especially in chip design) has been increasingly and presently known as “Neuromorphic Engineering”. This is considered a sub-field of Electrical Engineering.
Jumping forward to present-day research and development, “neuromorphic computing” implies the promise of a processing of data in more of an analog manner rather than the digital manner traditionally known in our daily computers. It could, for instance, imply the implementation of artificial neural networks onto a computer chip. This, for instance, could mean that the intensity of the signal is not bluntly on or off (read: 1 or 0) but rather that there could be a varying intensity. One could read more in relation to this and some forms of artificial neural networks by, for instance, looking at gates, thresholds, and the practical application of the mathematical sigmoid function; to name but a few.
Simultaneously, these later years, following 1988 until about 1995, some claim, can be referred to as the second surge of an “AI Winter”.[34] Some seem to put the period a few years earlier.[35] The accuracy of years put aside, resources became temporarily limited again. Research and its output was perceived to be at a low. Concurrently, one might realize that this does not imply that all research and developments in both computing hardware and software halted during these so-called proverbial winters. The work continued, albeit for some with some additional constraints or under a different name or field (not as “AI”). One might agree that in science, research and development across academic fields seems to ebb, flow and meander yet, persist with grit.
From 1990 onward slowly, but surely, the concept of probability and “uncertainty” took more of the center-stage (i.e. Bayesian networks). Statistical approaches became increasingly important in work towards AI methods. “Evolution-based methods, such as genetic algorithms and genetic programming” helped to move AI research forward.[36] It was increasingly hypothesized that a learning agent could adapt to (or read: learn from) the changing attributes in its environment. Change implies the varying higher probabilities of a number of events occurring and the varying lower probability of some other attributes, as events, occurring.
AI solutions started to extract patterns from data sets rather than be guided by a line of code only. This probabilistic approach in combination with further algorithmic developments was gradually heralding a radically different approach from the previous “Knowledge-based Systems’. This approach to AI solutions has warranted in what some refer to as the AI Spring[37] some perceive the past few years up till present day.[38]
In the twenty-first century to present-day, Artificial Neural Networks have been explored in academic research and this with increasing success. More and more, it became clear that huge data sets of high quality were needed to make a specific area of research in AI, known as Machine Learning, more powerful.
During the first decade of this century Professor Li Feifei oversaw the creation of such a huge and high quality image dataset, which would be one of the milestones in boosting confidence back into the field of AI and the quality of algorithm design.[39]
This story now arrived at the more recent years of AI Research and Development.
Following the first decade of this twenty-first century, an increasing usage of GPUs (graphical processing units) as hardware to power Machine Learning applications could be seen. Presently, even more advanced processing hardware is suggested and applied.
Special types of Machine Learning solution are being developed and improved upon. Specifically Deep Learning appeared on the proverbial stage. The developments in the Artificial Neural Networks and the layering of these networks become another important boost in the perception of potentials surrounding applications coming out of AI Research and Development (R&D).[40]
Deep Learning is increasingly becoming its own unique area of creative and innovative endeavor within the larger Field of AI.
Globally, major investment (in the tens of billions of dollars) have been made into AI R&D. There is a continued and even increasing hunger for academics, experts and professionals in various fields related to or within the field of AI.
The
historical context of the field of AI, of which the above is a handpicked
narrative, has brought us to where we are today. How this research is applied
and will be developed for increased application will need to be studied, tried
and reflected on, with continued care, considerate debate, creative spirit and
innovative drive.
Footnotes & URLs to Additional Resources
[1] Olazaran, M. (1996). A Sociological Study of the Official History of the Perceptrons Controversy. Social Studies of Science, 26(3), 611–659. London: Sage Publications
[2] Here loosely based on: Professor Dan Klein and Professor Pieter Abbee. (January 21st, 2014) CS188 “Intro to AI” Lecture. UC Berkeley.
[3] George Boole (1815 – 1864) came up with a kind of algebraic logic that we now know as Boolean logic in his works entitled The Mathematical Analysis of Logic (1847) and An Investigation of the Laws of Thought (1854). He also explored general methods in probability. A Boolean circuit is a mathematical model, with calculus of truth values (1 = true; 0 = false) and set membership, which can be applied to a (digital) logical electronic circuitry.
[4] McCulloch, W.. & Pitts, W. (1943; reprint: 1990). A Logical Calculus of the Ideas Immanent in Nervous Activity. Bulletin of Mathematical Biophysics, Vol. 5, pp.115-133. Retrieved online on February 20, 2020 from https://www.cs.cmu.edu/~./epxing/Class/10715/reading/McCulloch.and.Pitts.pdf
[5] Turing, A.M. (1950). Computing Machinery and Intelligence. Mind 49: 433-460. Retrieved November 13, 2019 from http://cogprints.org/499/1/turing.html and https://www.csee.umbc.edu/courses/471/papers/turing.pdf
[6] Spice, B. (April 11, 2017). Carnegie Mellon Artificial Intelligence Beats Chinese Poker Players. Online: Carnegie Mellon University. Retrieved January 7, 2020 from https://www.cmu.edu/news/stories/archives/2017/april/ai-beats-chinese.html
[7] Martinez, E. (2019). History of AI. Retrieved on April 14, 2020 from https://historyof.ai/snarc/
[8] Minsky, M. (2011). Building my randomly wired neural network machine. Online: Web of Stories Retrieved on April 14, 2020 from https://www.webofstories.com/play/marvin.minsky/136;jsessionid=E0C48D4B3D9635BA883747C9A925B064
[9] Russell, S. and Peter Norvig. (2016) and McCorduck, Pamela. (2004). Machines Who Think. Natick, MA.: A K Peters, Ltd.
[10] McCarthy, J. (2007). What is AI? Retrieved on December 5th, 2019 from http://www-formal.stanford.edu/jmc/whatisai/node1.html This webpage also offers a nice, foundational and simple conversation about intelligence, IQ and related matters.
[11] McCorduck, P. (2004). Machines Who Think: A Personal Inquiry into the History and Prospects of Artificial Intelligence. Natick: A K Peters, Ltd
[12] Rosenblatt, F. (January, 1957). The Perceptron. A Perceiving and Recognizing Automaton. Report No. 85-460-1. Buffalo (NY): Cornell Aeronautical Laboratory, Inc. p. 1 & 30 Retrieved on January 17, 2020 from https://blogs.umass.edu/brain-wars/files/2016/03/rosenblatt-1957.pdf
[13] Popper, K. (1959, 2011). The Logic of Scientific Discovery. Taylor and Francis
[14] Minsky, M. and Papert, S.A. (1971). Artificial Intelligence Progress Report. Boston, MA:MIT Artificial Intelligence Laboratory. Memo No. 252. pp. 32 -34 Retrieved on April 9, 2020 from https://web.media.mit.edu/~minsky/papers/PR1971.html or http://bitsavers.trailing-edge.com/pdf/mit/ai/aim/AIM-252.pdf
[15] Minsky, M. and Papert, S.A. (1969, 1987). Perceptrons: An Introduction to Computational Geometry. Cambridge, MA: The MIT Press
[16] Olazaran, M. (1996). A Sociological Study of the Official History of the Perceptrons Controversy. Social Studies of Science, 26(3), 611–659. London: Sage Publications
[17] Samuel, A.L. (1959, 1967, 2000). Some Studies in Machine Learning Using the Game of Checkers. Online: IBM Journal of Research and Development, 44(1.2), 206–226. doi:10.1147/rd.441.0206 Retrieved February 18, 2020 from https://dl.acm.org/doi/10.1147/rd.33.0210 and https://www.sciencedirect.com/science/article/abs/pii/0066413869900044 and https://researcher.watson.ibm.com/researcher/files/us-beygel/samuel-checkers.pdf
[18] It is known as the “unification algorithm”. Robinson, John Alan (January 1965). A Machine-Oriented Logic Based on the Resolution Principle. J. ACM. 12 (1): 23–41 Retrieved on March 24, 2020 from https://dl.acm.org/doi/10.1145/321250.321253 and https://web.stanford.edu/class/linguist289/robinson65.pdf
[19] The form is what now could be referred to as a logic-based declarative programming paradigm = the code is telling a system what you want it does and that by means of formal logic facts and rules for some problem and not exactly by stating how step by step it needs to do it. There are at least 2 main paradigms with each their own sub-categories. This logic-based one is a subcategory of the declarative programming set of coding patterns and standards. The other main paradigm (with its subsets) is imperative programming which includes object-oriented and procedural programming. The latter includes the C language. See Online: Curlie Retrieved on March 24, 2020 from https://curlie.org/Computers/Programming/Languages/Procedural Examples of (class-based) object-oriented imperative programming languages are C++, Python and R. See: https://curlie.org/en/Computers/Programming/Languages/Object-Oriented/Class-based/
[20] Minsky, M. and Papert, S.A. (1969, 1987) p. 231 “Other Multilayer Machines”.
[21] Lighthill, Sir J. (1972). Lighthill Report: Artificial Intelligence: A General Survey. Retrieved on April 9, 2020 from http://www.chilton-computing.org.uk/inf/literature/reports/lighthill_report/p001.htm and https://pdfs.semanticscholar.org/b586/d050caa00a827fd2b318742dc80a304a3675.pdf and http://www.aiai.ed.ac.uk/events/lighthill1973/
[22] Bostrom, N. (2014). Superintelligence. Paths, Dangers, Strategies. Oxford: Oxford University Press. p. 22
[23] McCorduck, P. (2004). pp. xxviii – xxix
[24] Minsky, M. and Papert, S.A. (1969, 1987)
[25] Historic Examples: Pierce, J. R. et al (1966). Language and Machines: Computers in Translation and Linguistics. Washington D. C.: The Automatic Language Processing Advisory Committee (ALPAC). Retrieved on April 9, 2020 from The National Academies of Sciences, Engineering and Medicine at https://www.nap.edu/read/9547/chapter/1 alternatively: http://www.mt-archive.info/ALPAC-1966.pdf
[26] Hutchins, W. J. (1995). Machine Translation: A Brief History. In Koerner, E. E.K. .et al (eds). (1995). Concise history of the language sciences: from the Sumerians to the cognitivists. Pages 431-445. Oxford: Pergamon, Elsevier Science Ltd. p. 436
[27] Russell, S. et al. (2016, p.24) doesn’t seem to mention this first “AI Winter” and only mentions the later one, by the end of the 1980s nor does McCorduck, Pamela. (2004) pp. xxviii – xxix. Ghatak, A. (2019, p. vii) however, identifies more than one, as do Maini, V., et al. (Aug 19, 2017) and Mueller, J. P. et al. (2019, p. 133), Chollet, F. (2018). P12 Perhaps these authors, who are mainly focusing on Deep Learning, see the absence of research following the Rosenblatt’s perceptron as a “winter”.
[28] Goodfellow, I., et al. (2016, 2017). Deep Learning. Cambridge, MA: The MIT Press. p. 2
[29] More in-depth information can be found in the journal of the same name: https://www.journals.elsevier.com/knowledge-based-systems
[30] Hutchins, W. J. (1995). p. 436
[31] Some Prolog resources related to expert systems: https://www.metalevel.at/prolog/expertsystems AND https://en.wikibooks.org/wiki/Prolog
[32] McCarthy, J. (1996). Some Expert Systems need Common Sense. Online: Stanford University, Computer Science Department. Retrieved on April 7, 2020 from http://www-formal.stanford.edu/jmc/someneed/someneed.html
[33] Mead, C. Information Retrieved on April 8, 2020 from http://carvermead.caltech.edu/ also see Mead, C. (1998). Analog VLSI and Neural Systems. Reading, MA: Addison-Wesley from https://dl.acm.org/doi/book/10.5555/64998
[34] Russell (2016) p. 24
[35] McCorduck (2004) p. 418
[36] Bostrom, N. (2014). Superintelligence. Paths, Dangers, Strategies. Oxford: Oxford University Press. pp.24
[37] Manyika, J. et al (2019). The Coming of AI Spring. Online: McKinsey Global Institute. Retrieved on April 9, 2020 from https://www.mckinsey.com/mgi/overview/in-the-news/the-coming-of-ai-spring
[38] Olhede, S., & Wolfe, P. (2018). The AI spring of 2018. Significance, 15(3), 6–7. Retrieved on April 9, 2020 from https://rss.onlinelibrary.wiley.com/doi/epdf/10.1111/j.1740-9713.2018.01140.x
[39] Deng, J. et al. (2009). ImageNet: A Large-Scale Hierarchical Image Database. Online: Stanford Vision Lab, Stanford University & Princeton University Department of Computer Science. Retrieved April 7, 2020 from http://www.image-net.org/papers/imagenet_cvpr09.pdf
[40] Trask, A. W. (2019). Grokking Deep Learning. USA: Manning Publications Co. p. 170